|
Econometric Modeling of Time Series: Forecasting and Analyzing Dynamic Data Training Course
USD 3,000 |
Venue: Nairobi
Other Dates
Venue | Date | Fee | |
---|---|---|---|
Nairobi, Kenya | 14 - 25 Apr, 2025 | USD3000 | |
Mombasa, Kenya | 14 - 25 Apr, 2025 | USD3500 | |
Nairobi, Kenya | 05 - 16 May, 2025 | USD3000 | |
Dubai, United Arab Emirates | 12 - 23 May, 2025 | USD5500 | |
Nairobi, Kenya | 19 - 30 May, 2025 | USD3000 | |
Nairobi, Kenya | 02 - 13 Jun, 2025 | USD3000 | |
Mombasa, Kenya | 09 - 20 Jun, 2025 | USD3500 | |
Nairobi, Kenya | 16 - 27 Jun, 2025 | USD3000 | |
Nairobi, Kenya | 07 - 18 Jul, 2025 | USD3000 | |
Johannesburg, South Africa | 14 - 25 Jul, 2025 | USD5500 |
Time series data, which tracks observations over time, is crucial for understanding and predicting a wide range of economic and financial phenomena. This Econometric Modeling of Time Series training course provides you with the advanced skills needed to effectively model, forecast, and interpret complex time series data. You'll learn sophisticated statistical and econometric methods, including ARIMA modeling, volatility analysis, cointegration, and advanced forecasting techniques, enabling you to extract valuable insights and make data-driven decisions in dynamic environments. This course bridges the gap between theoretical econometrics and practical application, providing you with in-demand skills for analyzing time-dependent data.
Target Audience:
This course is designed for professionals and researchers who work with time series data and require advanced analytical skills. The target audience includes:
- Economists and forecasters
- Financial analysts and risk managers
- Statisticians and data scientists
- Researchers in social sciences, environmental science, and other fields dealing with time series data
- PhD students in relevant disciplines
- Anyone seeking to master econometric time series techniques
Course Objectives:
Upon completion of this Econometric Modeling of Time Series training course, participants will be able to:
- Understand the properties of stationary and non-stationary time series.
- Master ARIMA modeling, including model identification, estimation, and diagnostic checking.
- Apply advanced forecasting techniques, such as exponential smoothing and dynamic regression models.
- Model and forecast time-varying volatility using ARCH and GARCH models.
- Analyze long-run relationships between time series using cointegration techniques.
- Implement advanced time series methods using statistical software (e.g., R or Stata).
- Interpret and communicate time series analysis results effectively.
- Evaluate the performance of different time series models and forecasts.
- Understand the limitations and assumptions of various time series techniques.
- Apply time series analysis to real-world problems in economics, finance, and other fields.
Duration: 10 Days
Course Content
Module 1: Introduction to Time Series Analysis
- What is a time series? Examples and applications in economics, finance, and other fields.
- Key concepts: Time series data, stationarity, autocorrelation, seasonality, trend.
- Objectives of time series analysis: Forecasting, modeling, and understanding patterns.
- Course overview: Structure, learning objectives, software tools (R or Stata), and assessment methods.
Module 2: Fundamentals of Time Series
- Stationarity: Strict and weak stationarity, testing for stationarity (e.g., ADF test, KPSS test).
- Autocorrelation and autocovariance: Measuring and interpreting relationships within a time series.
- Partial autocorrelation: Identifying the order of autoregressive models.
- Transformations: Dealing with non-stationarity (e.g., differencing, logarithmic transformations, Box-Cox transformations).
Module 3: ARIMA Modeling I: Model Identification
- Autoregressive (AR) models: Properties, estimation, and interpretation.
- Moving average (MA) models: Properties, estimation, and interpretation.
- ARMA models: Combining AR and MA components.
- ACF and PACF plots: Identifying the order of AR and MA components.
- Model identification strategies.
Module 4: ARIMA Modeling II: Estimation and Diagnostics
- ARIMA models: Extending ARMA models to non-stationary data (integrated component).
- Model estimation: Maximum likelihood estimation (MLE).
- Diagnostic checking: Residual analysis, Ljung-Box test, checking for normality.
- Model selection: AIC, BIC, and other information criteria.
Module 5: Forecasting with ARIMA Models
- Forecasting process: Point forecasts and interval forecasts.
- Forecast evaluation: Accuracy measures (e.g., RMSE, MAE, MAPE).
- Forecasting horizons: Short-term, medium-term, and long-term forecasting.
- Practical examples: Forecasting economic and financial time series.
Module 6: Advanced Forecasting Techniques
- Exponential smoothing: Holt-Winters and other methods for trend and seasonality.
- Dynamic regression models: Incorporating explanatory variables into time series models.
- State space models: Kalman filter and smoothing for complex time series.
- Applications: Forecasting with complex time series patterns.
Module 7: Volatility Modeling I: ARCH Models
- Volatility: Definition, importance, and stylized facts of financial time series volatility.
- Autoregressive conditional heteroscedasticity (ARCH) models: Modeling time-varying volatility.
- Properties and estimation of ARCH models.
- Testing for ARCH effects.
Module 8: Volatility Modeling II: GARCH Models
- Generalized ARCH (GARCH) models: Extending ARCH models to allow for persistence in volatility.
- GARCH model extensions: EGARCH, TGARCH, and other variations.
- Volatility forecasting: Using GARCH models to predict future volatility.
- Applications: Risk management, option pricing, and portfolio optimization.
Module 9: Cointegration and Error Correction Models
- Cointegration: Long-run equilibrium relationships between time series.
- Testing for cointegration: Engle-Granger test, Johansen test.
- Error correction models (ECM): Modeling short-run dynamics and long-run equilibrium.
- Applications: Modeling relationships between financial assets, macroeconomic variables, and other economic time series.
Module 10: Time Series Regression and Causality
- Regression with time series data: Dealing with non-stationarity and serial correlation.
- Spurious regression: The dangers of regressing non-stationary variables.
- Cointegration regression: Regressing cointegrated variables.
- Testing for Granger causality.
- Applications: Modeling relationships between economic and financial variables.
Module 11: Multivariate Time Series Analysis
- Vector autoregressive (VAR) models: Modeling multiple time series.
- Vector error correction models (VECM): Extending VAR models to cointegrated data.
- Impulse response analysis and forecast error variance decomposition.
- Applications: Forecasting macroeconomic variables, financial market analysis, and other multivariate time series.
Module 12: Advanced Topics and Applications
- Seasonality: Modeling and forecasting seasonal time series.
- Intervention analysis: Assessing the impact of events on time series.
- Time series analysis in specific fields: Economics, finance, environmental science, and other relevant areas.
- Emerging trends in time series analysis: Machine learning and deep learning for time series forecasting.
Training Approach
This course will be delivered by our skilled trainers who have vast knowledge and experience as expert professionals in the fields. The course is taught in English and through a mix of theory, practical activities, group discussion and case studies. Course manuals and additional training materials will be provided to the participants upon completion of the training.
Tailor-Made Course
This course can also be tailor-made to meet organization requirement.
Training Venue
The training will be held at our Skills for Africa Training Institute Training Centre. We also offer training for a group at requested location all over the world. The course fee covers the course tuition, training materials, two break refreshments, and buffet lunch.
Visa application, travel expenses, airport transfers, dinners, accommodation, insurance, and other personal expenses are catered by the participant
Certification
Participants will be issued with Skills for Africa Training Institute certificate upon completion of this course.
Airport Pickup and Accommodation
Airport pickup and accommodation is arranged upon request.
Terms of Payment: Unless otherwise agreed between the two parties’ payment of the course fee should be done 5 working days before commencement of the training.
Course Booking
Please use the “book now” or “inquire” buttons on this page to either book your space or make further enquiries.
Nairobi | Apr 07 - 18 Apr, 2025 |
Nairobi, Kenya | 14 - 25 Apr, 2025 |
Mombasa, Kenya | 14 - 25 Apr, 2025 |
Nairobi, Kenya | 05 - 16 May, 2025 |
Dubai, United Arab Emirates | 12 - 23 May, 2025 |
Nairobi, Kenya | 19 - 30 May, 2025 |
Nairobi, Kenya | 02 - 13 Jun, 2025 |
Mombasa, Kenya | 09 - 20 Jun, 2025 |
Nairobi, Kenya | 16 - 27 Jun, 2025 |
Nairobi, Kenya | 07 - 18 Jul, 2025 |
Johannesburg, South Africa | 14 - 25 Jul, 2025 |
USD 3,000.00 | |
Nixon Kahuria +254 702 249449
Tags: |
Time Series Analysis Econometrics Forecasting Dynamic Data Statistical Modeling Regression Analysis ARIMA Machine Learning Financial Econometrics Data Analytics |
Related Courses

12 days, 07 - 18 Apr, 2025
Skills for Africa Training Institute

12 days, 07 - 18 Apr, 2025
Skills for Africa Training Institute

5 days, 07 - 11 Apr, 2025
Skills for Africa Training Institute
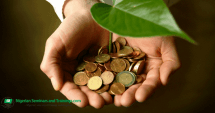
5 days, 07 - 11 Apr, 2025
Skills for Africa Training Institute
Currency Converter